Real MOS devices are affected by the generation, transformation and charging of oxide and interface defects. The complex interplay of these physical processes leads to a shift of the threshold voltage in response to changes of the gate bias which results in hysteresis and bias-temperature instability (BTI). Experimentally, for larger stress times, one often observes a recoverable BTI component, caused by charging of pre-existing oxide defects, and a quasi-permanent BTI component with long recovery times. The latter component is typically ascribed to defect generation, although the underlying physical mechanisms are less understood (see the discussion in
It has been shown that the recoverable component can be accurately captured using a 2-state non-radiative multi phonon (NMP) model. The quasi-permanent component, on the other hand, can be approximately described using a simple double-well (DW) model with a field dependent energy barrier, at least at the present level of understanding. In this section we will add both defect models to our device to lay the foundation for the subsequent BTI simulation.
- For donor-like traps, state 1 is neutral and state 2 is positively charged.
- For acceptor-like traps, state 1 is negatively charged and state 2 is neutral.
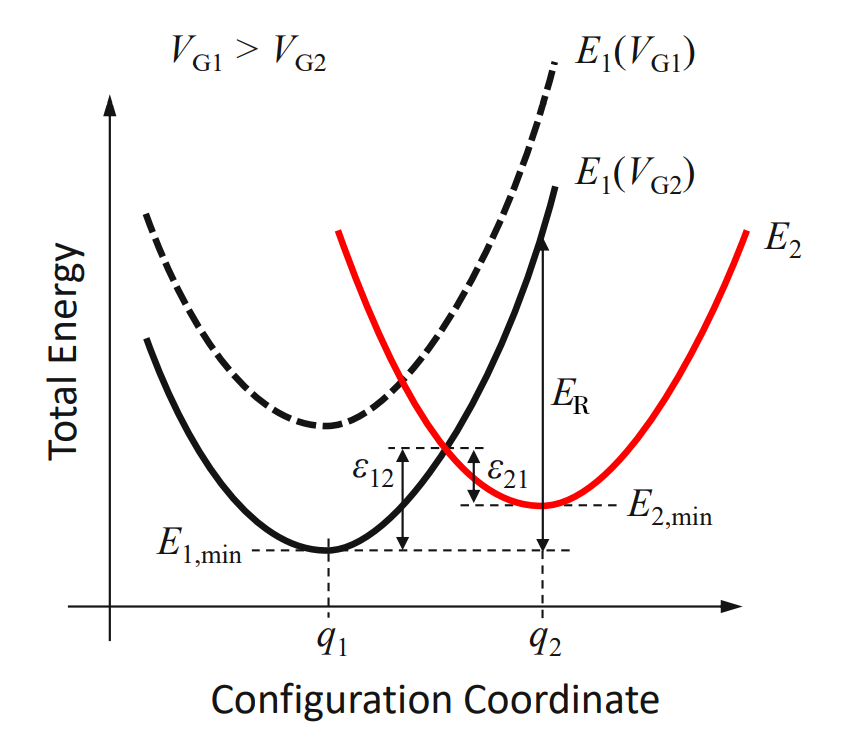
In order to simulate the threshold voltage shift \(\Delta V_\mathrm{th} \) caused by a (partially) charged defect ensemble we have to specify the underlying distribution of the defect parameters. The choice most frequently found in the literature is a normal distribution for the energetic parameters \(E_\mathrm{T}\), \(E_\mathrm{R}\) and \(R\), while the spatial positions \( x_\mathrm{T} \) of the defects are usually assumed to be uniformly distributed, resulting in a constant defect density in a given area of the oxide. This choice is referred to as Gaussian defect band and has been used successfully to reproduce experimental data across a large variety of different devices. However, in exemplary studies the distribution of \(R\) was found to be narrow, hence, \(R\) is approximated as a constant in the Comphy framework. Consequently, each Gaussian NMP defect band can be characterized by the following 9 parameters:
parameter | Comphy notation | description | unit |
---|---|---|---|
\(\langle E_\mathrm{T} \rangle \) | Et | mean energy level | eV |
\( \sigma_{E_\mathrm{T}} \) | Et_sigma | standard deviation of energy levels | eV |
\(\langle E_\mathrm{R} \rangle \) | Er | mean relaxation energy | eV |
\( \sigma_{E_\mathrm{R}} \) | Er_sigma | standard deviation of relaxation energies | eV |
\( x_\mathrm{T,min} \) | xt_min | lower bound of spatial defect distribution | m |
\( x_\mathrm{T,max} \) | xt_max | upper bound of spatial defect distribution | m |
\( R \) | R | curvature ratio of defects | 1 |
\( N_\mathrm{T} \) | Nt | defect concentration of defect band | m⁻³ |
- - | trap_type | type of the traps (either donor or acceptor) | - - |
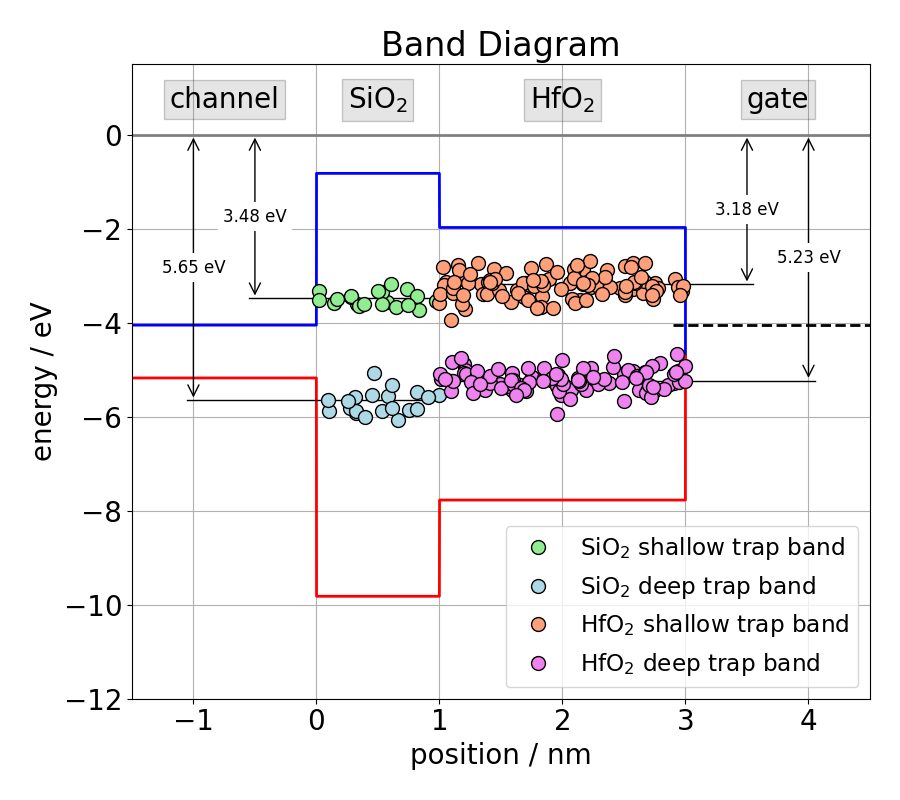
defect band name | x_start | x_end | Et | Et_sigma | Er | Er_sigma | R | Nt | trap_type |
---|---|---|---|---|---|---|---|---|---|
SiO2_shallow | 0.0E-9 | 1.0E-9 | -3.48 | 0.15 | 3.82 | 1.36 | 0.407 | 1.5e25 | 'acceptor' |
SiO2_deep | 0.0E-9 | 1.0E-9 | -5.65 | 0.24 | 2.90 | 1.67 | 1.01 | 5.42E25 | 'donor' |
HfO2_shallow | 1.0E-9 | 3.0E-9 | -3.18 | 0.16 | 3.19 | 0.77 | 0.59 | 9.05E25 | 'acceptor' |
HfO2_deep | 1.0E-9 | 3.0E-9 | -5.23 | 0.15 | 3.55 | 0.741 | 1.01 | 5.16E25 | 'donor' |
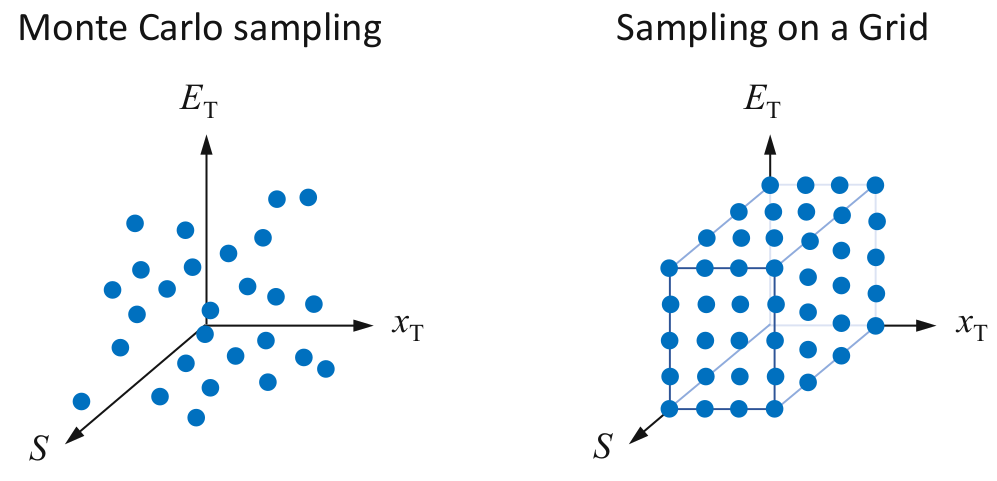
|
|
|
|
|
|
|
|
|
|
|
|
|
|
|
|
|
|
|
|
|
|
|
|
|
|
|
|
|
|
my_device.trap_bands['SiO2_shallow_trap_band'] = SiO2_shallow_trap_band
SiO2_deep_trap_band = TrapBand_2S_NMP(
|
|
|
|
|
|
|
|
|
|
|
|
|
|
|
|
|
|
|
|
|
|
my_device.trap_bands['SiO2_deep_trap_band'] = SiO2_deep_trap_band
HfO2_shallow_trap_band = TrapBand_2S_NMP(
|
|
|
|
|
|
|
|
|
|
|
|
|
|
|
|
|
|
|
|
|
|
my_device.trap_bands['HfO2_shallow_trap_band'] = HfO2_shallow_trap_band
HfO2_deep_trap_band = TrapBand_2S_NMP(
|
|
|
|
|
|
|
|
|
|
|
|
|
|
|
|
|
|
|
|
|
|
my_device.trap_bands['HfO2_deep_trap_band'] = HfO2_deep_trap_band
- Generation and annealing of oxide defects
- Activation and passivation of dangling bonds
- Transformation of existing oxide defects (H relocation)
- Metastability of pre-existing oxide defects
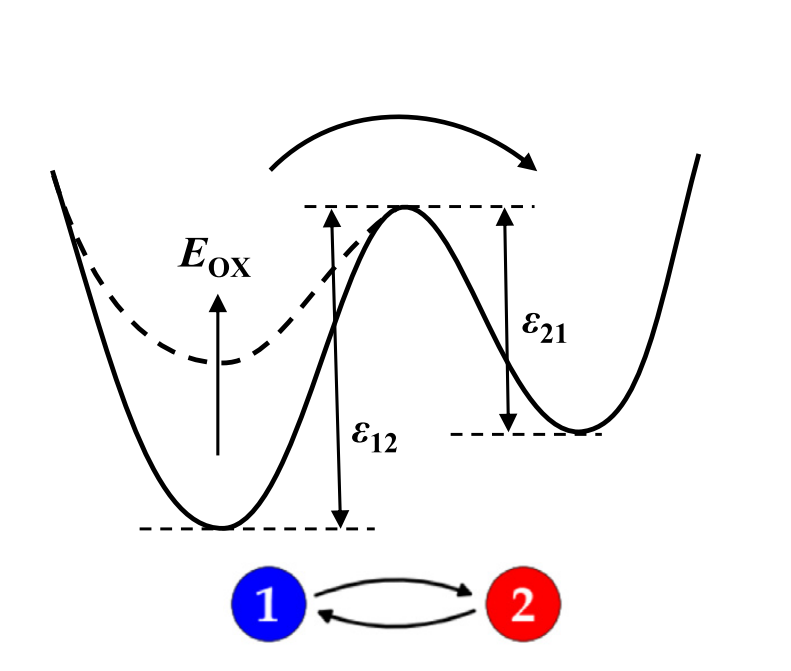
Similar to the Gaussian NMP trap band, we assume \(\epsilon_{12,0}\) and \(\epsilon_{21}\) to be normally distributed while the other parameters are approximated as constant. Consequently, a Gaussian DW defect band can be described in the Comphy framework by the following 8 parameters:
parameter | Comphy notation | description | unit |
---|---|---|---|
\(\langle \epsilon_{12,0} \rangle \) | eps1 | mean activation energy barrier | eV |
\( \sigma_{\epsilon_{12,0}} \) | eps1_sigma | standard deviation of activation energy barriers | eV |
\(\langle \epsilon_{21} \rangle \) | eps2 | mean passivation energy barrier | eV |
\( \sigma_{\epsilon_{21}} \) | eps2_sigma | standard deviation of passivation energy barriers | eV |
\( k_0 \) | k0 | transition rate for zero barrier | s⁻¹ |
\( \gamma \) | gamma | empirical field dependence of activation barrier | eV m V⁻¹ |
\( N_\mathrm{t} \) | Nt | interface defect concentration | m⁻² |
-- | trap_type | type of traps (either donor or acceptor) | -- |
defect band name | eps1 / eV | eps1_sigma / eV | eps2 / eV | eps2_sigma / eV | k0 / s | gamma / eV m V⁻¹ | Nt / m⁻² | trap_type |
---|---|---|---|---|---|---|---|---|
interface_traps | 2.5297 | 0.92612 | 1.649 | 0.060114 | 1.2336E+13 | 1.7052E-10 | 1.9096E+17 | donor |
|
|
|
|
|
|
|
|
|
|
|
|
|
|
|
|
|
|
|
|
|
|
|
|
my_device.initialize(
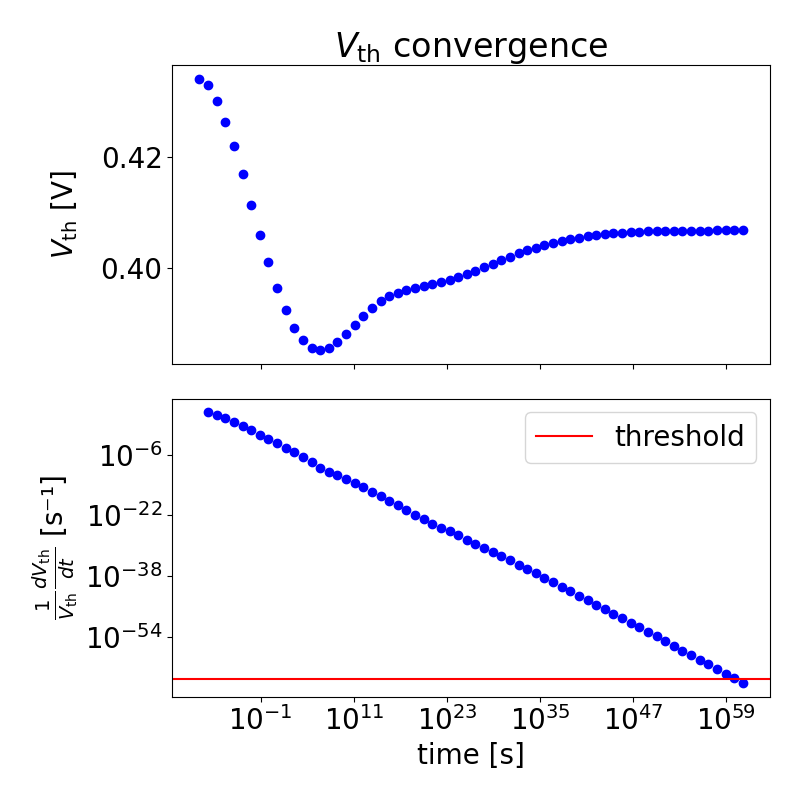
plot_band_diagram(my_device)
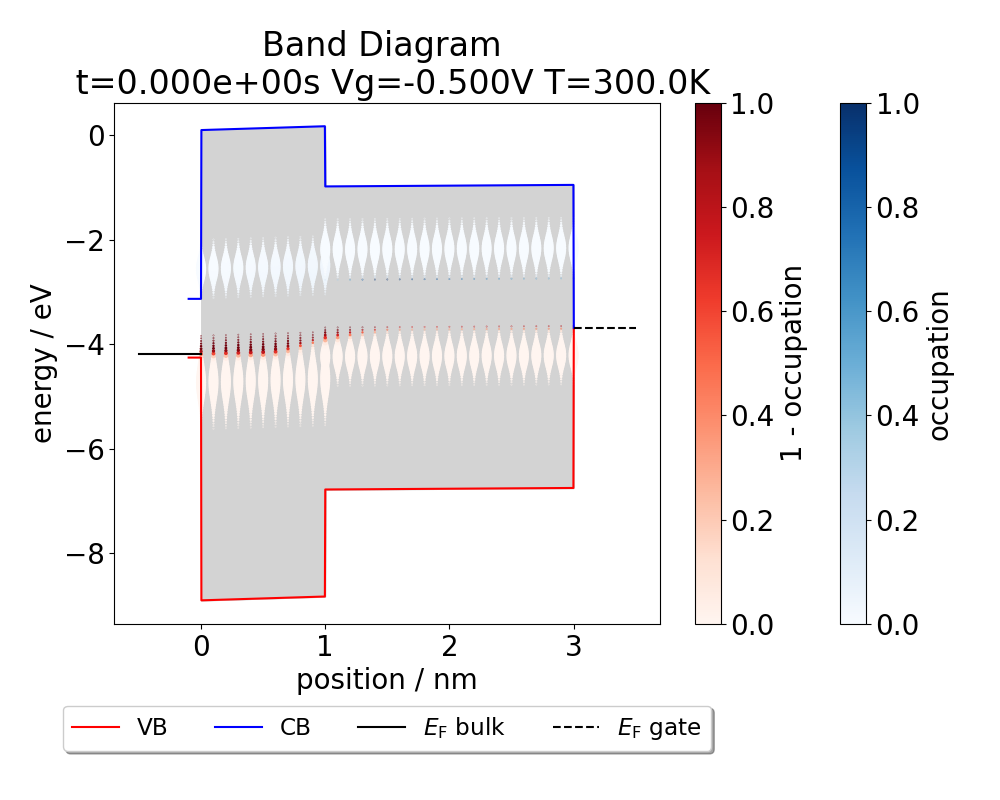